Project Title: A Physics-Based Artificial Intelligence General Framework for Optimal Control of Sewer Systems to Minimize Sewer Overflows
Funding Agency: U.S. National Science Foundation
Period: October 2022 - September 2025
PI: Arturo S. Leon
Co-PI: Mohammadhadi Amini
Project Summary:
The project will bridge big data computational algorithms with physics-based models to create the first integrated and general physics-based AI model to predict sewer overflows and mitigate them through an optimal sequence of decision variables at control gates. The proposed research converges multi-disciplinary knowledge and tools such as lab-scale overflow experiments, CFD modeling, reduced-order sewer modeling, hydrological modeling, and ML to advance our understanding of sewer overflow mitigation. The primary outcomes of the proposed research will include (1) a new sewer overflow module for an existing open-source sewer flow dynamics model (ITM); (2) a new hydraulic engine (ITM) for the widely used Storm Water Management Model (SWMM); and (3) an integrated open-source and general physics-based AI model for predicting sewer overflows for a given fixed set of controls (e.g., gate positions are fixed) and for determining an optimal sequence of decision variables at control gates for minimization of sewer overflows.












Sequential snapshots in the horizontal pipe of one of our geyser experiments
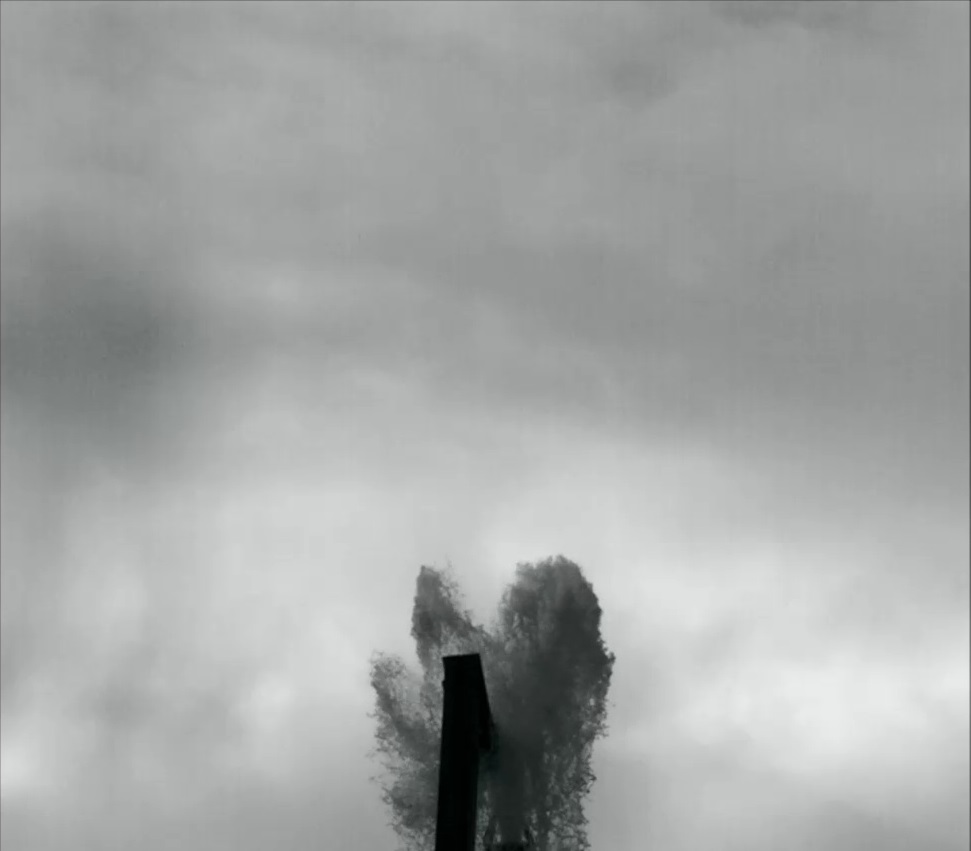
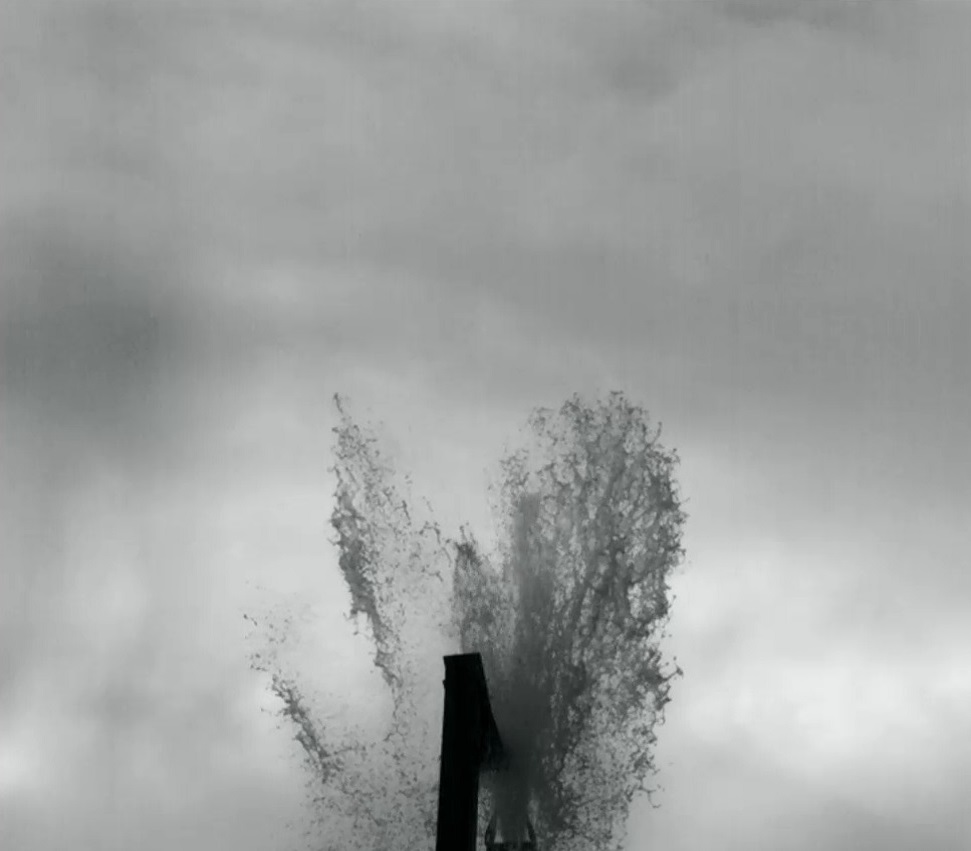
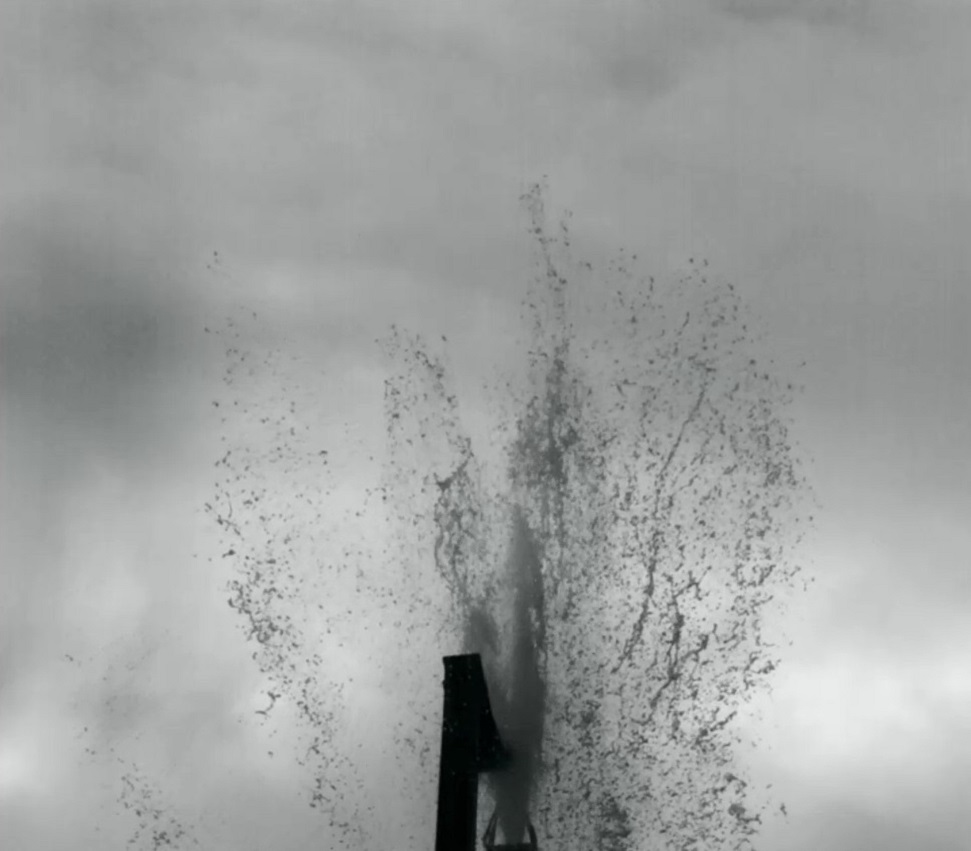
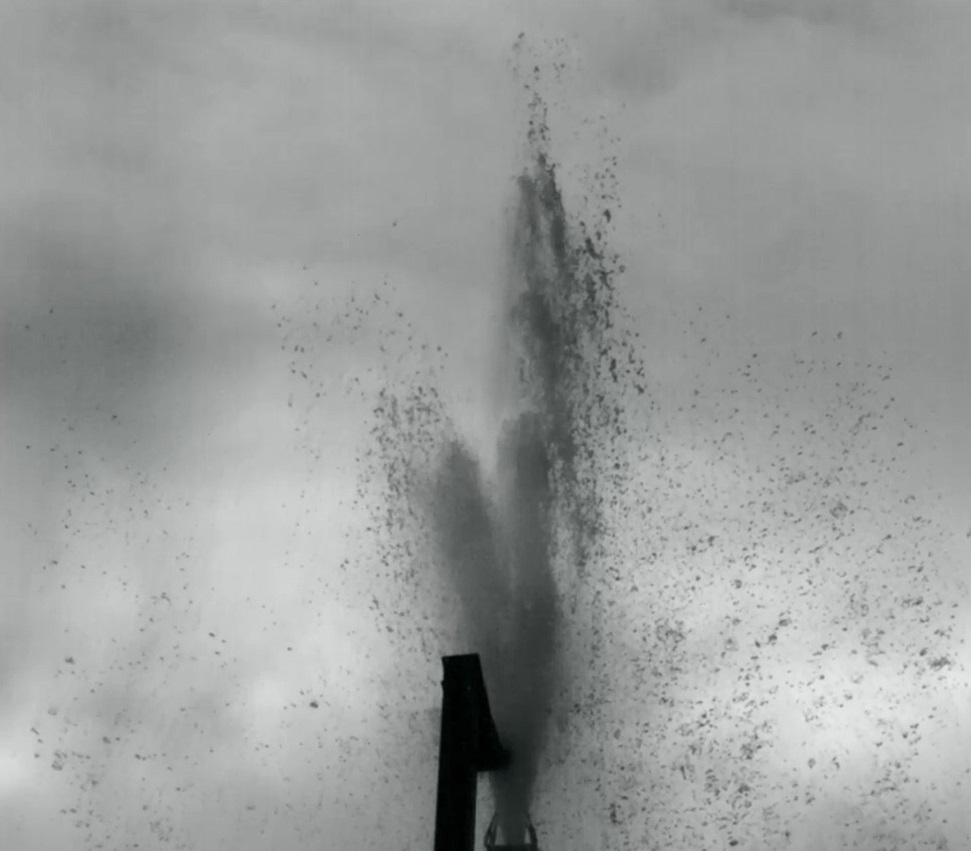
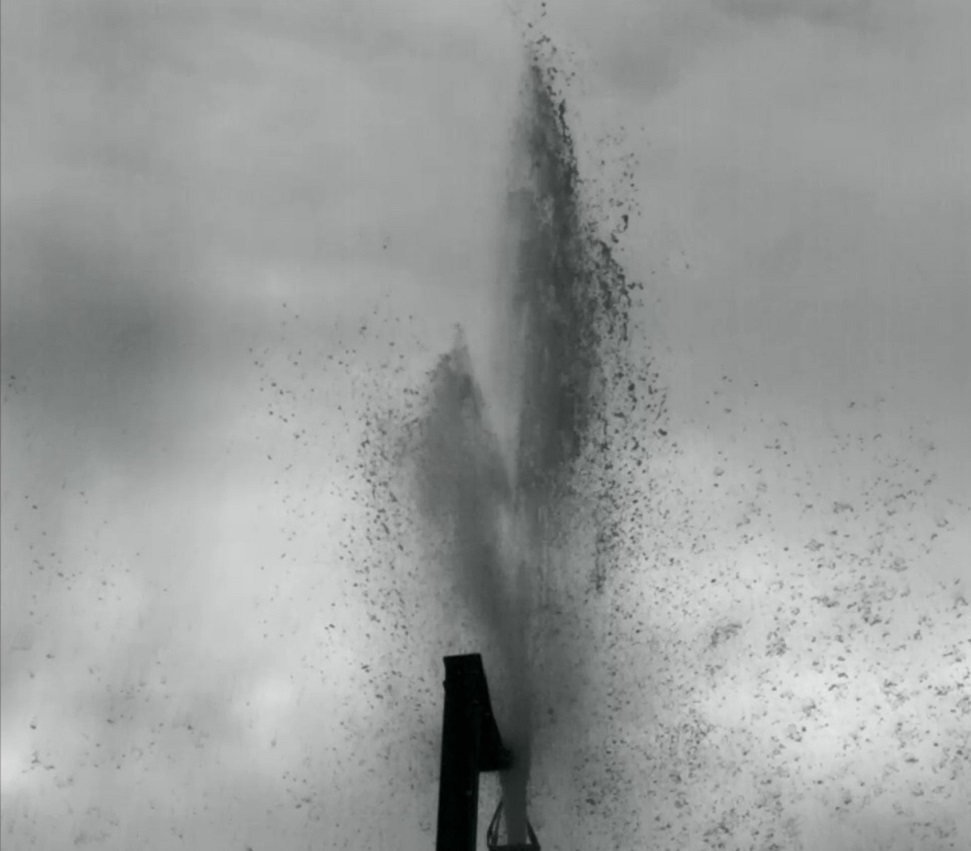
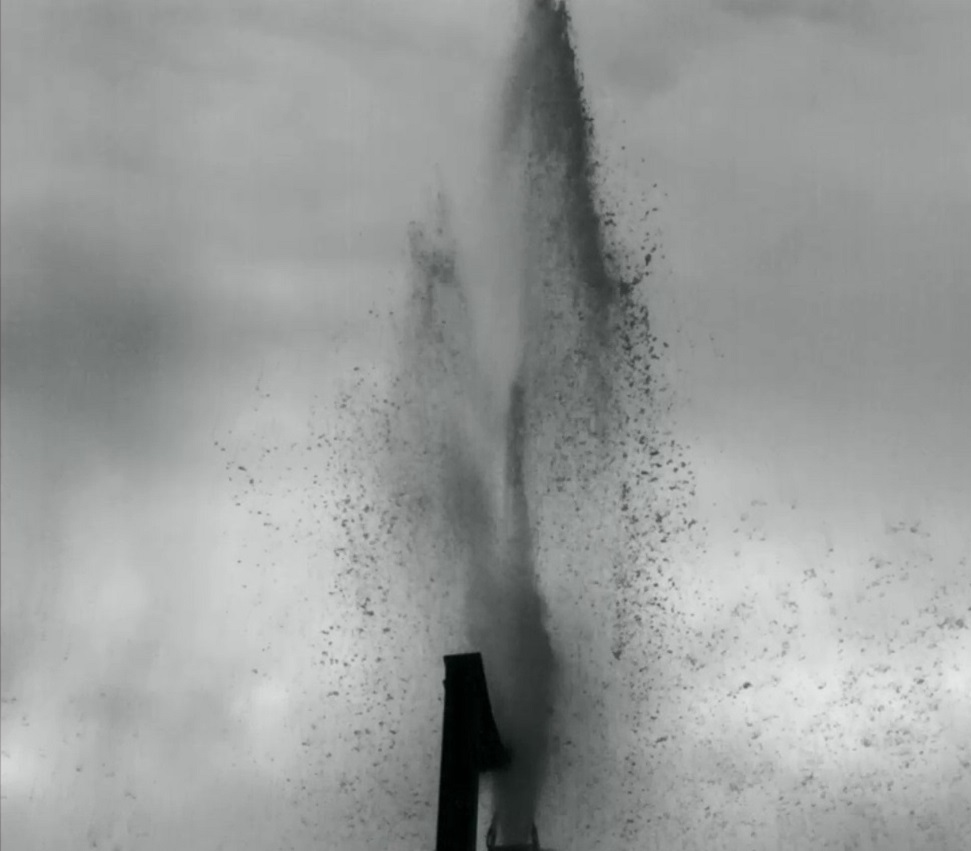
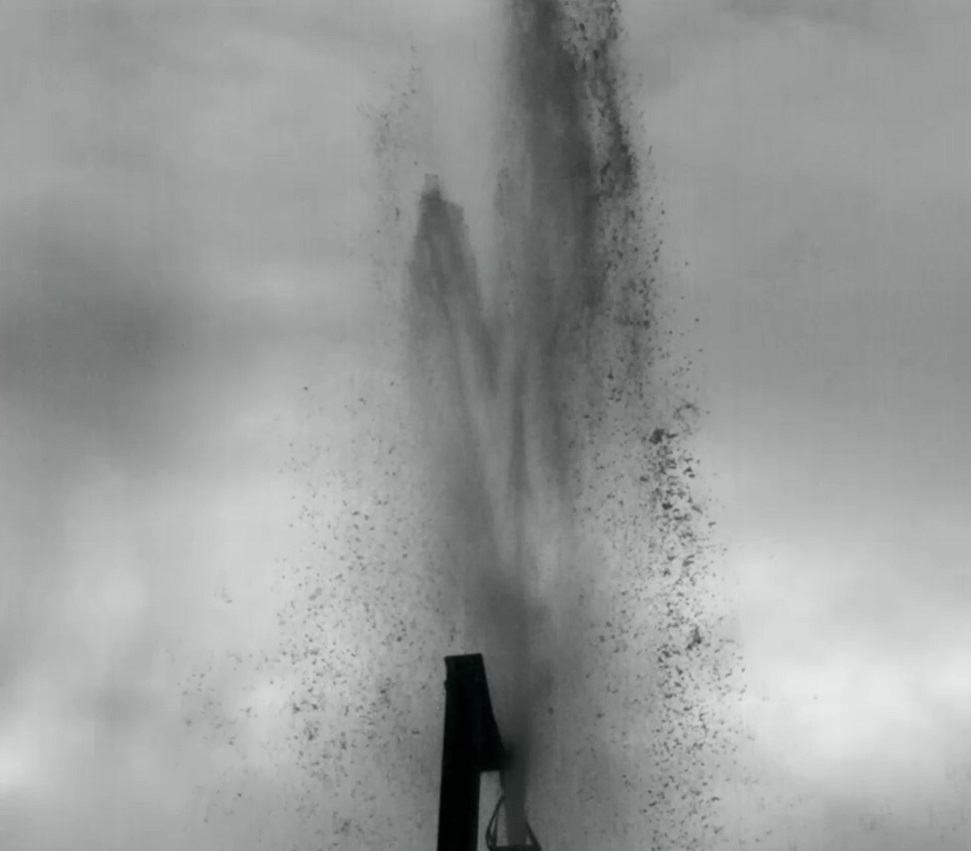
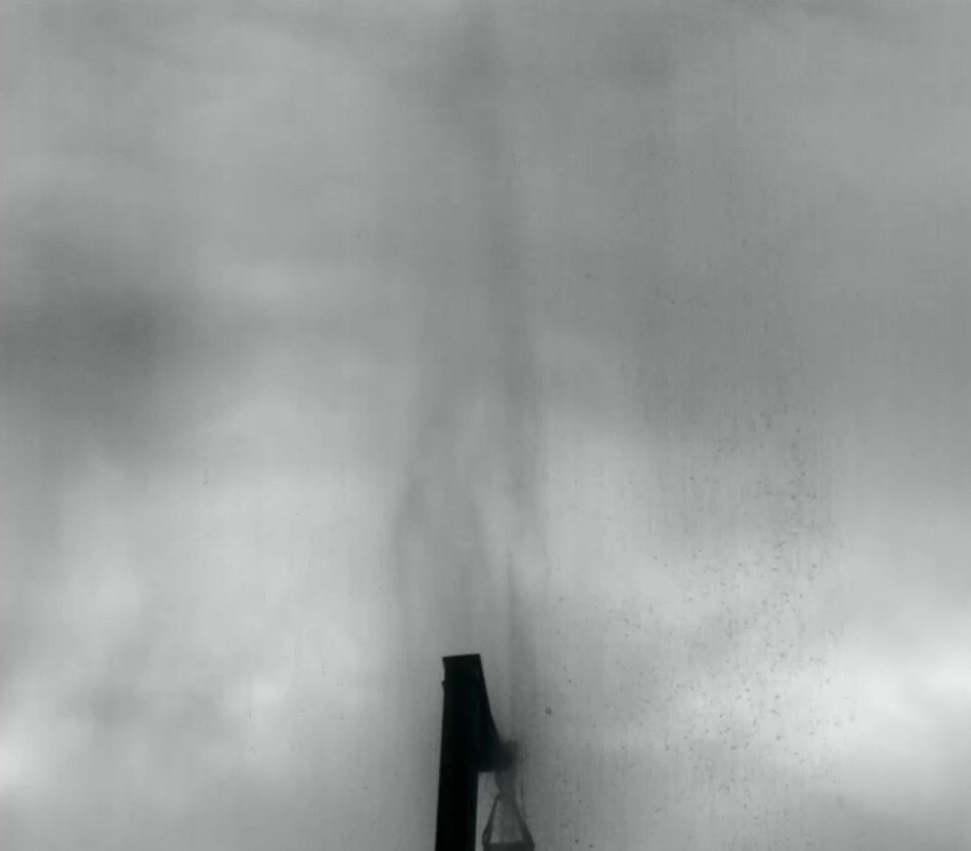
Sequential snapshots of geyser eruption in one of our experiments
Combined Sewer Overflows (CSOs) pose a threat to public health and the environment, contributing to contamination of drinking water, beach closures, and other concerns. Predicting ahead of heavy rainfalls when and where sewer overflows are likely to occur and the sequence of decisions to minimize overflows require an ultrafast integrated modeling framework. Artificial intelligence (AI) via machine learning (ML) is gaining popularity for guiding sewer systems' operation. A critical obstacle for implementing ML in sewer systems is the long period (~ 5 years) of detailed field data needed to produce reliable results. Even if the sewer system is monitored for a few years, machine learning based on limited field data cannot anticipate projected climate change scenarios. Instead of field data, this project aims to use numerical simulations for ML training. The project's overall goal is to bridge big data algorithms, lab-scale experiments, and physics-based numerical models to accelerate the availability of ML models for the optimal operation of sewer systems to reduce sewer overflows. The specific aims are to (R1) implement and validate a sewer overflow model (ITM-OVER) for an existing open-source sewer flow dynamics model (ITM) that currently cannot simulate sewer overflows; (R2) develop a general physics-based AI open-source framework for predicting the location and volume of CSOs for a given fixed set of controls (e.g., gate positions are fixed); and (R3) develop an AI open-source framework for determining an optimal sequence of decision variables at control gates for minimization of CSOs. For aim R1, we will develop ITM-OVER and validate it using lab experiments and Computational Fluid Dynamics (CFD) modeling. For aims R2 and R3, we will first develop a general ML model in PyTorch, a Python open-source ML framework. Then, various open-source physical-based models and our ML model will be integrated into a framework named IMPACTO. Then, IMPACTO will be trained to (1) predict the location and volume of CSOs in two existing sewer systems and (2) determine the optimal sequence of decision variables at control gates (e.g., flow discharges) for minimization of sewer overflows. The accuracy of IMPACTO will be evaluated utilizing scenarios that were not used in the ML training. The main educational objective is to increase underrepresented students' participation in undergraduate engineering programs. To this end, hands-on activities on sewer overflows will be offered in an existing outreach program targeted primarily to underrepresented middle-school students.
Project Participants:
Current Research Outcomes:
This project started on August 01, 2022. For the research outcomes of this project, please click the buttons below accordingly.
Website visitors since January 30, 2020
COPYRIGHT, ARTURO S. LEON